Predicting and Preventing Crime: An Interview with CTDS’s Dr Roman Marchant
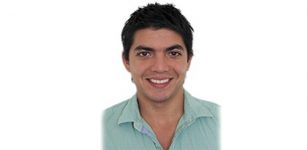
The future is now. Even before you move into your new model home, in that recently-designed outer suburb in metropolitan Sydney, big data is watching you. And it doesn’t need to monitor your movements, or even address your motives, it just needs to know your postcode.
Or that’s one way you could perceive the new developments in crime research that are taking place at the Centre for Translational Data Science (CTDS) at the University of Sydney.
A recently completed research project into “hidden patterns in criminal activity” has utilised historical crime data and applied statistical modelling and machine learning to achieve outcomes whereby predictions about crime levels can be made by analysing certain socioeconomic factors
Australia a family/domestic violence nightmare
The initial project has taken aim at a scourge that causes terrible harm throughout the community: domestic violence. A household terrorism that everybody wants to see the end of.
In Australia, an average of one woman is killed every week as a result of this type of violence. Last year, 71 women were killed in domestic violence situations across the country. And around 650 family violence matters are reported to police each day nationwide.
One in three Australian women over the age of 15 will experience some form of violence at the hands of a person at home in their lifetime. And many believe that police often don’t take these types of reports seriously enough.
Statisticians preventing further violence
A team of Sydney University researchers, led by data scientist Dr Roman Marchant, has been analysing domestic violence statistics for all regions of NSW at the SA2 level – areas that contain about 2,000 to 20,000 people – for about a year now.”
Not surprisingly, researchers have found areas of high population density, greater unemployment and large numbers of men that are separated from their partners, have higher levels of domestic violence.
And this data is of value, as it can be utilised to prevent further crime.
As Dr Marchant outlines below, the team’s research informs long-term governmental policies and short-term policing programs which are designed to curb domestic violence levels within the community.
But big data is really watching
The researchers have the main objectives, as the doctor pointed out, of reducing crime and improving the wellbeing of society. And for the moment, they’re collecting data on large groups of people and deducing what crime levels can be expected judging from past experience.
However, certain mathematical algorithms are being utilised, along with machine learning – artificial computerised intelligence – to come to conclusions about criminal behaviour out there in the community.
And they hope that as they progress, they can incorporate time variables into their methods, so that future crime levels – sometimes in neighbourhoods that don’t even exist as yet – can be predicted.
Sydney Criminal Lawyers® spoke with Dr Roman Marchant, who also researches in the Sydney Institute of Criminology, about the juxtaposition between social science and data research that can provide cutting edge ways of understanding criminal behaviours.
So the purpose of your data study was to gain greater understanding of crime: why, when and where it occurs. What were the areas you looked at during your study, both regionally and crime-wise? And what were the key socioeconomic indicators you took into consideration?
The area we have data for is the entire state of New South Wales. And in each area, our resolution is at the SA2 level: statistical area level two. These are smaller than LGAs – local government areas – and have between 2,000 and 20,000 people in each. So we can predict crime and understand crime at each one of those individual cell levels.
So that’s the area and the resolution.
And the factors that we’re considering are firstly, total household income, mortgage repayments and weekly rent. So that’s the first one we trial: economic factors.
Then people with no religion is another one of the variables that we’ve considered. So separating people that are religious from non-religious people. Then the number of males that are separated. The median age of the population in an area and the unemployment percentage.
Then also the population of the area, or the population density. People that were born outside of Australia. Then English-speaking only people. And then education levels. So the number of people that have completed a certification. And then single-parent families.
So these are the variables. And if it’s income, then it’s a median value. If it’s a mortgage, it’s a median mortgage.
We’ve come up with this shortlist of variables after speaking with a number of people from institutes of criminology, experts on criminology and sociology as well, who have told us what they think these are the variables that can explain a particular type of crime.
Now the type of crime that we’re analysing, in this case, is domestic violence.
So currently these experiments that we’ve done focus on domestic violence. But our model is general enough so it can try to explain the occurrence of any type of crime, however, we have to analyse these separately.
So you apply statistical modelling and machine learning -a type of artificial intelligence that provides computers with the ability to learn without being explicitly programmed – to historical crime data to find the patterns in criminal activity. How do you go about applying this sort of approach practically?
So the first thing that we have to do is get crime data and at the same time we get the data from the demographics – from the Census data, in this case.
So we gather all the data. Then we do an exploratory analysis, which is really looking at rough trends and how crime is changing all the time. How much of the different crime types we have. And then we identify if there are clusters of crime at different locations, just by exploring the data.
Then we clean the data. We remove crimes that aren’t really of interest. We select the crime type and the most interesting variables.
Then we place a model. We define a mathematical representation of what crime could be. And that part takes quite a long time and a lot of expertise from mathematical statisticians. So I’m a statistician myself, but then I work with professors that have a lot of experience modelling.
Then you say, “I’m applying a probabilistic model.” It’s important to note here that these aren’t deterministic models. So we’re not saying, “There’s going to be an exact number of crimes. We know how everything is going to happen.”
But all of our models are probabilistic, which means that we provide an estimation of crime levels, with an associated uncertainty.
We derive these models that allow us to relate the risk and protective factors to the actual number of crime occurrences at each location. And then the most important part I would say – together with these derivations of the mathematical models – is how do we learn the parameters of these models?
Because what happens is that you can define a model, but this model has free parameters, which is the tuning that allows this model to fit a specific data set.
And that’s where the machine learning comes into play. Learning patterns means learning these parameters and how these general models can fit a specific phenomenon, which in this case is domestic violence in NSW.
So we need to learn what the parameters are that help us explain the occurrence of crime, and that’s where machine learning comes into play.
So it’s a good relationship between statistical modelling and math, and machine learning, which pretty much implements the algorithms that allows us to learn the model.
What’s the purpose of gathering this crime data? Can this data be used in a preventative way?
Our ultimate goal is to be able to reduce crime and improve the wellbeing of society. That’s our main focus.
And for that you obviously need to be able to make long-term policy decisions to address issues in society that foster criminal activity, or in the short-term to be used by police forces to be able to allocate resources, such as where to open a new police station, or how many patrolling units for domestic violence at certain times of the day in specific areas.
So we have short-term and long-term possibilities of using these models to reduce crime.
We’re also considering in the future doing research into other specific areas, so particularly my area of expertise is sequential decision making under uncertainty – so how you can make optimal decisions to maximise your rewards.
But that’s another area we’re hoping to explore in the future. How police can make decisions, or how governments can make decisions in order to reduce crime. But in order to do that efficiently you must first understand the problem – the phenomena.
So that’s what we’re tackling. That’s our first goal is to understand why crime is happening and where it’s happening. And then we can start planning and doing research on how to make decisions.
So I’ve got a couple of questions about your findings. In what areas do you expect to find higher levels of criminal activity? Does say population density affect the rates of crime? And besides that, what are the other key drivers of crime?
There’s an important thing here that I have to mention. There’s certain unified relations that I’m going to be exploring now.
For each individual factor, the model can either express whether it either positively or negatively affects crime in a positive or negative way, because it’s like a risk factor or a protective factor.
You can look at each one of these effects individually, but that doesn’t represent necessarily the whole picture, because it’s a multivariable problem, so it’s a combination of all these factors that gives rise to a specific type of crime. And not each one individually.
That’s actually the good thing in criminology, is that we learn everything in a multivariate and joint model.
Now if you look at them separately you get some insight into what’s causing crime, but you need to have a look at the combination of them.
But anyway, if we talk about a specific variable, so let’s say, for example, you mentioned population density, in this case, the population of an area positively affects crime. Which means that if you have more people packed into an area, there’s more crime happening.
And this is one of the most important factors actually.
We did this study several times. For crimes happening in 2001, and for crimes around 2006 and 2011, which are the Census dates. And all our results are consistent within these three sections of the data. So population density has a large effect.
And then we have other variables that positively affect crime as equally important as population density, and one is the number of separated males. So if you have a lot of separated males you expect more domestic violence, which makes total sense.
Do you mean separated or single males?
Separated.
Separated from their partners?
Yes, where their marriage has come to an end.
So population per area, if you have a more densely packed area and you have domestic violence, then it’s likely to be reported by neighbours, because it’s denser, so they can hear the couple fighting and usually they would call the police.
That’s the most usual case, so that’s why you’d expect that to happen. It’s good that the results are reflecting that.
Then you have separated males, which of course, leads to more fights or more disputes. There’s a reason why they separated to start with.
And then the next variable that is of importance is unemployment, because it introduces instability and financial stress into a household, which leads to domestic violence according to experts. So unemployment is the other big one that we found.
And then equally affecting crime in a positive way, but not as important as the previous ones we have, is English-speaking only.
It has a similar relation with people who are born somewhere else, so a birthplace elsewhere than Australia, and the reason for this is, we think, because usually immigrants, who don’t have for example a permanent visa, would definitely avoid getting in trouble with police because of their status in the country.
That’s one of the reasons we think that might be happening, but it’s not as important as unemployment or the number of separated men.
Then there are another set of variables that negatively affect crime. So if you pay more rent for example, then you would expect less domestic violence in these areas, than where people pay less rent.
And it’s the same case for income and with mortgage repayments.
So I was going to ask, would you say your research reveals that there’s a certain type of individual who’s more likely to pursue a life of crime? Or are these other factors more influential on an individual’s decision to pursue criminal activities?
All the results that we’re getting at the moment are over aggregated data, so we’re not looking at individual pathways at the moment, but we will. We’re already getting data about that.
But you really cannot conclude anything about individuals with this data. This is about aggregated parts of the population and they definitely help in explaining crime. But if you want to have a look at conclusions about individuals or how different life events or socioeconomic factors affect them, then you would have to do that kind of analysis yourself.
It’s not something that I could say with the results that we’re currently getting.
OK but what about young offenders? Did you come across any information about what sort of young people were becoming involved in a life of crime?
The results that we’re currently getting are that the age of the population is negatively related with crime.
So if you have older people living in an area, then you would expect less domestic violence and with the younger the more.
That’s something very well-known for criminologists nowadays, that young people between the age of 18 and 24 that’s where you have the highest peak in criminal activity.
And as for domestic violence, in our case, we found an area that has a median age of around 20 to 30 has much more domestic violence crime, than when the average age of the people that live in an area is much older.
So the older the people, the less domestic violence.
We definitely want to look at individual behaviour, but this is aggregated data. So the importance is that we can predict not for an individual person, but for an area.
What we did for our study is remove from our data – from the known data – some areas of Sydney.
Some that we randomly chose from different regions, and we said, “OK. We only know from these random regions the income, how much people pay for rent, how many males are separated and how much unemployment there is in the area.”
We tried using our model to guess the number of crimes that actually happened in those areas. And the models came up with pretty good predictions, which means that our model is fitting and generalising the data.
So if you come up with a new area, and you say this is a combination of factors. This is how unemployment will be, this is how many single-parent families there will be, along with other factors, we can actually achieve a prediction of how much crime you would predict in that area.
So that’s our most important result from this research.
So you’d be talking about a new suburb that’s going to be built in a certain area. And the government says, “We’re going to build a new suburb. These type of people will probably live here. We’ll have these sorts of institutions and this sort of infrastructure set up.” You’re saying that when one of these new suburbs is being built you’ll be about to have a probable prediction of what kind of crime levels to expect?
Yeah, we’d be able to predict it, but with probability distribution. I prefer that to prediction. You would have an expected value and variance as well.
So that’s actually one of the big projects that we developed this model for. Because you know in Camden the population is expected to rise around 200 percent by 2036, which it’s the largest forecasted percentage increase in any LGA in Australia.
And the idea is that we want to be able to use these models to assess what the crime levels would be in Camden in the near future by 2022 or something.
So we would be doing something exactly as you say. Looking at the mortgage that people who live there will pay, and how much income they will have, how much they will be paying for rent, depending on the housing. And the sort of people that will live there in the terms of income and employment etc. And try to estimate what the crime levels would be like.
What about the role of authorities, or say police in different areas? Does the level of policing, or the actual visual presence of police on the street deter the levels of criminal activity in an area?
That’s another big one. We haven’t been able to look at it as yet, but it’s another one of the big projects that we’re looking at, which is, what is the relation of the presence of police and actual criminal activity?
Interestingly enough, what the rough numbers say is that if you get more police in an area, instead of deterring crime, you find more. But that makes sense, as well, because you’re catching more criminals, so you’re finding more crime that is actually already hidden.
In that sense, it’s actually an unknown area and it’s not completely solved yet.
So we’re looking at getting data from the Bureau of Crime Statistics and Research and working with police as well, so we can understand the relationship between the presence of police and crime.
But that’s something we haven’t included in this study.
People who become involved in the criminal justice system in Australia have quite high reoffending or recidivism rates.
In NSW, around 48 percent of offenders return to prison within two years of having been released.
What sort of factors did you find lead people to desist from committing further crimes, rather than reoffending?
Again this study did not look at individual behaviour.
We’re already getting data about the reoffending history of many individuals for domestic violence – particularly in this case – but that’s another separate piece of work.
In that piece of work, we would be looking at the characteristics of around 200 different features from individual people over time, and what their contact with the criminal justice system has been over time as well.
We want to be able to assess what people are more likely to reoffend, than others.
That’s what that particular project is about, but we haven’t conducted the final analysis on that yet, although, BOSCAR has done some studies on this area and have released reports.
And lastly, you’ve looked at data from different time periods and from different areas.
From looking at the changes over time can you predict what sort of changes in regards to domestic violence you expect in the future?
Whether you expect more domestic violence to be happening, or whether you expect the levels to go down?
We certainly can. I don’t have a number that I can say you should expect in the future. But that’s what our models are built for.
I would say the main limitation here is that the uncertainties would increase the further into the future you go.
Because we’re using characteristics from the population to predict crime. So our models depend on unemployment, depend on income, the rent and all these sorts of things that also change over time.
In order to achieve a quantitative idea of uncertainties overtime we would really need to understand how each one of these individual variables will behave in the future, so the prediction becomes a very tricky problem.
We haven’t really assessed that yet, so this is like a cross sectional approach, where we said, “In 2001 these were the parameters that the model has found. In 2006 and 2011 as well.”
Now it would be great and that’s what we’re doing for the next situation of the ongoing research is to explicitly include time as a variable in our model, which would allow us to say, “OK for 2016 if these are the values or the distributions for unemployment and population density in specific areas, this is the crime levels that we will have in the future.”
That’s what we’re planning to do.
But can you make some probable prediction about whether in the Sydney metropolitan area domestic violence is expected to rise or fall in the next five years say?
I think yeah we could do this kind of analysis, but it’s not something that I could report to you right now, because we haven’t done it. But this is one of the possible applications of the model.
Dr Marchant thanks very much for taking the time out to have this chat with us today. And best of luck with your future work delving into the world of crime.
Yeah great Paul. Thank you.